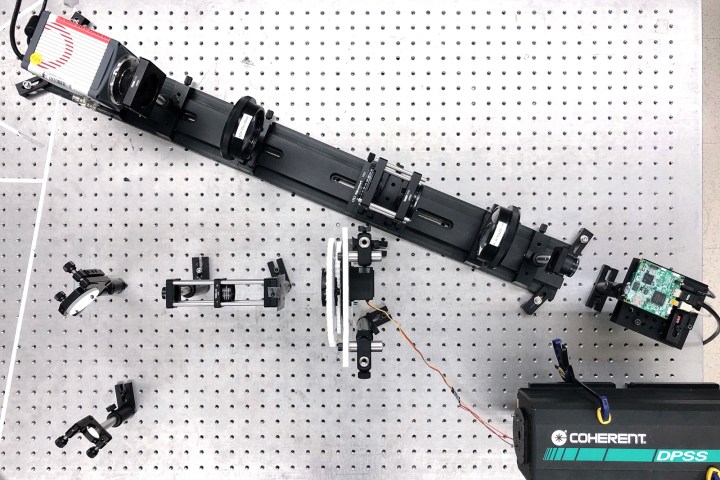
The camera is the eye for many automated devices and the computer is the brain — but researchers at Stanford University recently combined the two in an attempt to make smart cameras more compact. A team of graduate students recently created an artificially intelligent camera that doesn’t need a large, separate computer to process all the data — because it’s built into the optics itself.
Current object recognition technology uses A.I. on a separate computer to run the images or footage through algorithms to identify objects. As the Stanford researchers explain, driverless cars have a large computer in the trunk in order to recognize when a pedestrian steps out in front of the car’s path. Those computers are big, require lots of energy and are often slow.
The researchers instead worked to build the A.I. directly into the camera, both to create smaller systems as well as faster ones. The camera has two layers — the first is called an optical computer. As light passes through the camera, the built-in computer pre-processes that data. The optical computer filters out unnecessary data, reducing the number of necessary calculations. The second layer is a traditional computer and imaging sensor that handles the remaining calculations. The science behind the A.I. camera is complex, but the result could lead to significant advances for devices with built-in cameras like self-driving cars and drones.
By processing the scene as the light hits the camera instead of after the fact, the researchers said they were able to create a camera that didn’t require input power, reducing the typically intense computing power required for object recognition A.I. “We’ve outsourced some of the math of artificial intelligence into the optics,” graduate student Julie Chang said.
Gordon Wetzstein, the assistant professor that led the research, says that moving the computing to the optics allows for much faster data processing, along with reducing the computing required. “Millions of calculations are circumvented and it all happens at the speed of light,” he said.
While one of the goals is to reduce the size of different devices, the research hasn’t quite reached that point yet. The camera takes up a lab bench, but the researchers suggest that further research will help the concept to decrease in size, eventually making the AI camera small enough for more portable devices. The team suggests several different potential uses, from autonomous cameras and drones to handheld medical imaging. The processing at the speed of light could be a big advantage in self-driving cars that rely on cameras to see and avoid potential collisions.
The device’s size suggests the technology needs some time to mature before actually being integrated into actual products, but the research takes a significant step forward for artificially intelligent cameras. The team recently published the full research in Nature’s Scientific Reports.