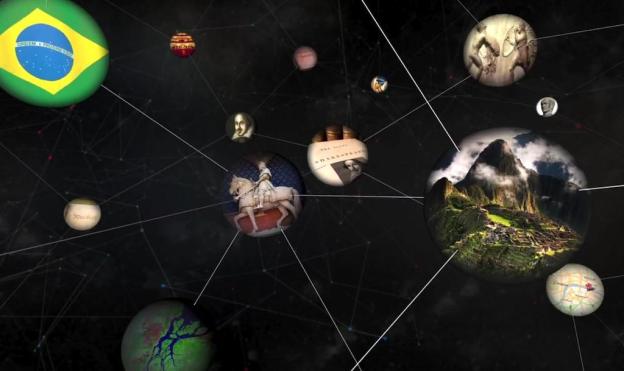
Google is starting to roll out its new Knowledge Graph technology to its English-speaking users in the United States. Although the new service will be popping up as an adjunct to Google’s normal Web search results — rather than a separate service in its own right — it represents a fundamentally different way to approaching search. Instead of returning ranked search results based on literal search terms (or some search terms, or possibly-corrected versions of some of search terms), Knowledge Graph essentially attempts to associate search queries with stuff it knows about: places, people, books, movies, events — you name it. Knowledge Graph is an effort to achieve semantic search, attempting to return results based on the meaning of what users search for, instead of just literal matches.
Can the Knowledge Graph change the way we search? And what might it mean for Google’s fundamental business — and sites that rely on Google to bring traffic to their sites?
Knowledge Graph under the hood
Although Knowledge Graph is a fundamentally new kind of search offering from Google, it follows well-trodden paths Google has been pursuing for years with its mainstream search service. And Google is being careful to introduce it in a way that isn’t terribly disruptive to its market-dominating search.
For years, Google has been able to answer a selection of simple factual queries directly from the search bar, and even do some math — handy for people who are more likely to have a Web browser running than a calculator. Try it: Google should provide direct answers to things like “capital of suriname” or “square root 3952.”
With Knowledge Graph, Google will also be dropping search queries into complex databases of interrelated information about…well, things, for lack of a better terms. In some ways these databases function much like a traditional lookup: they return records with important bits of information about a particular thing. For a person, that might be something like their birth date (and maybe death date), their nationalities, titles or offices they may have held, full legal name, and more.
For a building, these datasets might include things like its location, when it was built, its overall size, its type (say, monument, retail space, commercial space, residence, um…space station?). However, in addition to what amount to a few bare facts and some keywords, these database entries also collect together direct links to related objects in the database (which in turn link to other related objects, and so on). In all probability, the nature of those links are defined too. For instance, an entry around a person might contain links to that person’s parents, spouse(s), and children, and other significant relationships and be able to distinguish between family members and other types of relationships. The database wouldn’t be doing its job if an dataset on George H. W. Bush (the 41st President of the United States) didn’t link to dataset on George W. Bush (the 43rd President) — and both would link to Condoleezza Rice, but in different ways. A dataset on the Great Pyramid should include links to Cheops and Khufu, and The Sphinx — but also to the Mausoleum at Halicarnassus. (Can you guess why?)
These datasets make up the heart of semantic search — and they don’t come cheap. First of all, they’re huge: The sum of human knowledge may be but a tiny speck in the face of all the information in the universe, but just scraping the service can easy produce hundreds of millions (or billions) of datasets. (In comparison, the English version of Wikipedia has a scant 4 million or so articles.) These datasets aren’t easy to get: they have to be painstakingly compiled from reliable sources. Furthermore, they have to be organized and designed in such a way that the information can be accessed and manipulated in useful ways (and in real time, for Google’s purposes). And the datasets have to be able to cope with the maleable nature of “knowledge.” After all, just a few years ago, Pluto was a planet and Vioxx was an FDA-approved osteoarthritus treatment.
Google is apparently building its databases using technologies and methods acquired with Metaweb back in 2010 — although Metaweb’s Freebase semantic database remains available to anyone. Google is using Freebase for data, along with information culled from Wikipedia and the CIA World Factbook. Google claims its Knowledge Graph database already has entries for some 500 million objects (please note thee objects can’t be directly compared to Wikipedia articles) and some 3.5 billion “facts.” We put “fact” in quotes because it was once a “fact” that the Earth was flat and humans couldn’t fly. Knowledge is slippery.
Knowledge Graph on the screen
Google’s initial implementation of Knowledge Graph is designed to augment the company’s existing search results listings, rather than replace them. Much as Google sometimes shows previews of pages in a panel to the right side of search results in a standard Web browser window, Knowledge Graph results will appear in panels next to search results. Not all search terms will produce Knowledge Graph panels: Queries will have to match well-defined objects in the Knowledge Graph. (Don’t worry if you don’t see Knowledge Graph results just yet; Google is still rolling the feature out, and right now it’s limited to English-speaking users in the United States.)
The Knowledge Graph panels seek to display a summary of key and most-sought information about a query without requiring users to read through two-line summaries of a Web page or click through to another site. For a person, these key facts might include birth and death dates, significant people associated with them, and quick highlights of titles, accomplishments, or what else makes that person significant. For other entities, Google will try to surface key information, statistics, and associations. The Knowledge Graph panel will also handle disambiguation. If more than one Knowledge Graph entity matches a search query, Google provides access to them all.
Perhaps more significantly, once users are interacting with a Knowledge Graph entity they can, within some limits, surf the links of relationships to those entities. For instance, pulling up a Knowledge Graph entry on Dashiell Hammett ought to let users immediately jump to a Knowledge Graph summary of The Thin Man and The Maltese Falcon — and, perhaps to summaries about Lillian Helman and post-World War II anti-Communist witch hunts.
Knowledge Graph won’t be restricted to browser-based searches: Google is currently rolling out Knowledge Graph search results to most devices running Android 2.2 or higher (again, U.S.-only in English) in the Quick Search box and browser-based searchers. Knowledge Graph search results will also be introduced to forthcoming versions of Google’s search app for iOS devices. Users can navigate though information in Knowledge Graph by tapping or swiping back and forth through the content.
It’s important to note these are just the first places Knowledge Graph is surfacing in Google’s services. Behind the scenes, you can expect Knowledge Graph search results to begin informing a broad variety of Google services, particularly as its corpus of datasets and “facts” grows. Knowledge Graph searches will likely never replace Google’s traditional keyword-based search — semantic search and literal search are kind of two different tools good at two separate tasks — but, in theory, it wouldn’t be surprising if Knowledge Graph one day contributed to as much as a quarter of Google’s interactions with search users.
Crowdsourcing…or Google-colored classes?
So, how does Knowledge Graph pick information for its summaries? So far, Google hasn’t been very explicit about the methodology behind Knowledge Graph’s presentation. In my (limited) sampling, a good portion of the data Google prioritizes for its summaries seems to be pretty consistent: dates, relations, and a single “significant accomplishment” field for people (which could be labeled something like “Discoveries” or “Occupation” or “Title”). Places get locations and dates, and a selection of other fields that could be exactly what someone wants or completely inappropriate. For instance, if you’re looking at The Empire State Building, providing the street address seems appropriate…but it’s not quite as appropriate for, say, Stonehenge. Similar oddities can happen with phone numbers: how many people need instant access to a phone number for the Taj Mahal?
Google says it prioritizes the information it presents in Knowledge Graph summaries using “human wisdom.” And by that, Google doesn’t actually mean things that humans tell them or that subject experts or database curators collect — it means making indirect assumptions about users’ intentions by logging search behaviors and keeping tabs on what they click, don’t click, and look for after doing a search. In a nutshell, Google is using crowdsourcing to try to determine which “facts” are the best ones to present in a Knowledge Graph summary.
For example, Google says the Knowledge Graph summary information it presents for Tom Cruise answers 37 percent of Google search users’ follow-up queries about the actor when they search for him. That 37 percent number sounds re-assuringly scientific and precise, but there is absolutely no way to assess whether Google’s assessment of search users’ aggregate behavior has anything to do with what a particular user — like you — wants to know. Since Google seems so proud of that 37 percent figure, let’s turn it on its head: Google says 63 percent of the time, it can’t present any information about a topic that its search users find relevant.
Google’s position is easy to understand: Whenever possible, it wants to immediately present the information its users are seeking. The only way Google can really assess that is by looking at how people use its search engine and trying to do some guesswork.
Crowdsourcing has its dangers. Just as Google is treading in murky waters when it chooses to prioritize search results from Google+ in Search Plus Your World, there are hazards to relying on crowdsourcing to prioritize the presentation of information and “facts.” Just because Google’s search audience may not know (or particularly care) about certain information doesn’t mean it’s not important or relevant. There are plenty of cases where “the crowd’s” perception of facts are wrong. Most people think schizophrenia means having multiple personalities, drinking milk or eating ice cream increases mucus production, and Marie Antoinette said “Let them eat cake.” Yet none of these things are true.
Relying on crowdsourcing to assess the important of information also creates potential for abuse. Say a government wanted to seed misinformation about dissidents, a political campaign wanted to smear an opponent, or hackers wanted to play with search results just for laughs? In much the same way Google search results have been “Googlebombed,” crowdsourcing could be used to manipulate Knowledge Graph. Sensible people won’t believe everything they read; similarly, “facts” presented by semantic search engines will not be reliable — and in some cases crowdsourcing will make them even less so.
Making Google stickier
On the practical side, Google’s Knowledge Graph will have one immediate impact: It will make Google’s search results stickier. Whenever Knowledge Graph can provide a direct answer to a search user’s question — or let them navigate to it quickly via related topics — users will be staying on Google services. That means Google collects more data about users’ searches and behaviors (regardless of whether they’re signed in to a Google account or not). That, in turn, lets Google further refine its targeted advertising platform.
It also means that services like Wikipedia that often answer the same sorts of knowledge-specific queries targeted by Knowledge Graph will see a decline in the amount of Web traffic they receive from Google. In Wikipedia’s case, that directly corresponds to fewer opportunities to solicit community support; for other services, that will translate directly to a lower number of ad impressions and (hence) lower revenues. For folks who offer sites and services based on providing discrete facts and information — and that includes everything from Wikipedia to IMDb to online retailers to phone books and business directories to (conceivably) crowd-sourced services like Yelp and even public records…Knowledge Graph could slowly erode their businesses.