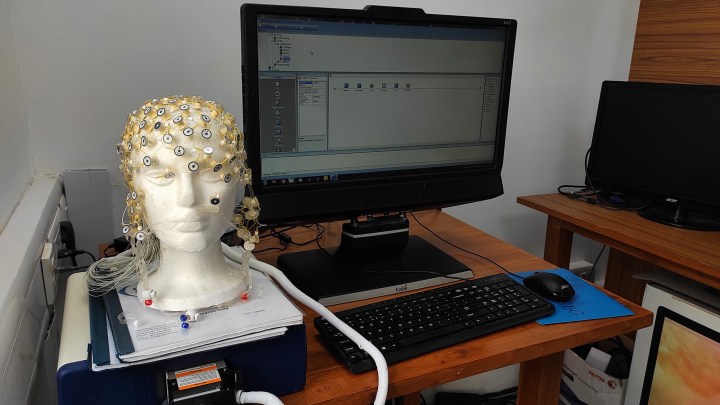
From Journey’s “Don’t Stop Believin’” to Queen’s “Bohemian Rhapsody” to Kylie Minogue’s “Can’t Get You Out Of My Head,” there are some songs that manage to successfully worm their way down our ear canals and take up residence in our brains. What if it was possible to read the brain’s signals, and to use these to accurately guess which song a person is listening to at any given moment?
That’s what researchers from the Human-Centered Design department at Delft University of Technology in the Netherlands and the Cognitive Science department at the Indian Institute of Technology Gandhinagar have been working on. In a recent experiment, they demonstrated that it is eminently possible — and the implications could be more significant than you might think.
For the study, the researchers recruited a group of 20 people. and asked them to listen to 12 songs using headphones. To aid with their focus, the room was darkened and the volunteers blindfolded. Each was fitted with an electroencephalography (EEG) cap that’s able to noninvasively pick up the electrical activity on their scalp as they listen to the songs.
This brain data, alongside the corresponding music, was then used to train an artificial neural network to be able to identify links between the two. When the resulting algorithm was tested on data it hadn’t seen before, it was able to correctly identify the song with 85% accuracy — based entirely on the brain waves.
“The songs were a mix of Western and Indian songs, and included a number of genres,” Krishna Miyapuram, assistant professor of cognitive science and computer science at the Indian Institute of Technology Gandhinagar, told Digital Trends. “This way, we constructed a larger representative sample for training and testing. The approach was confirmed when obtaining impressive classification accuracies, even when we limited the training data to a smaller percentage of the dataset.”
Reading minds, training machines
This isn’t the first time that researchers have shown that it’s possible to carry out “mind-reading” demonstrations that would make David Blaine jealous, all using EEG data. For instance, neuroscientists at Canada’s University of Toronto Scarborough have previously reconstructed images based on EEG data to digitally re-create face images stored in a person’s mind. Miyapuram’s own previous research includes a project in which EEG data was used to identify movie clips viewed by participants, with each one intended to provoke a different emotional response.
Interestingly, this latest work showed that algorithms that proved very effective at guessing the songs being listened to by one participant, after being trained on their specific brain, wouldn’t work so well when applied to another person. In fact, “not so well” is a gross understatement: The accuracy in these tests plummeted from 85% to less than 10%.
“Our research shows that individuals have personalized experiences of music,” Miyapuram said. “One would expect that the brains respond in a similar way processing information from different stimuli. This is true for what we understand as low-level features or stimulus-level features. [But] when it comes to music, it is perhaps the higher-level features, such as enjoyment, that distinguish between individual experiences.”
Derek Lomas, assistant professor of positive A.I. at Delft University of Technology, said that a future goal of the project is to map the relationship between EEG frequencies and musical frequencies. This could help answer questions such as whether greater aesthetic resonance is accompanied by greater neural resonance.
To put it another way, is a person who is “moved” by a piece of music going to show greater correlations between the music itself and the brain response, making it possible to accurately predict how much a person enjoys a piece of music simply by looking at their brain waves? While everyone’s response to music may be subtly different, this could help shed light on why humans seek out music to begin with.
The road to brain-computer interfaces
“For near-term applications [in the next two years], we imagine a music recommendation engine that could be based on a person’s brain response,” Lomas told Digital Trends. “I currently have a student working on algorithmically generated music that maximizes neural resonance. It’s quite eerie: The maximum neural resonance is not the same as maximum aesthetic resonance.”
In the medium term, Lomas suggested it could lead to powerful applications for gaining information on the “depth of experience” enjoyed by a person engaging with media. Using brain-analysis tools, it may (and, indeed, should) be possible to accurately predict how deeply engaged a person is while, say, watching a movie or listening to an album. A brain-based measure of engagement could then be used to hone specific experiences. Want to make your movie more engaging for 90% of viewers? Tweak this scene, change that one.
“In the long term — 20 years — this field of work may enable methods for transcribing the contents of the imagination,” Lomas continued. “For example, transcribing thoughts to text. That’s the big future of [brain-computer interfaces.]”
As Lomas noted, we are still a distance away from that eventual goal of a brain-computer interface. Nonetheless, work such as this suggests that there’s plenty of tasty lower-hanging fruit on that tree before we finally fell it.
A paper describing this research, titled GuessTheMusic: Song Identification from Electroencephalography, was recently presented at CODS-COMAD 2021.